Exploring Robust Optical Accelerators for Neural Networks
Master thesis
In recent years, deep neural networks (DNNs) have achieved remarkable breakthroughs. This advance, however, is accompanied by a rapid increase of network layers and operations in DNNs to improve the computation accuracy. Optical neural networks (ONNs) have emerged as a promising high-performance computing platform to accelerate DNNs. In ONNs, phases of light are modulated through Mach-Zehnder Interferometers (MZIs), and MZIs are connected in a grid-like layout to implement multiply-accumulate operations. Figure 1 and Figure 2 illustrate the structure of an MZI and an ONN structure with MZIs connecting together, However, ONNs are very sensitive to process variations and thermal effects. This sensitivity leads to a significant degradation of inference accuracy of ONNs and thus renders them unusable in practice. In this master thesis, countering hardware uncertainties with ensemble learning and architectural enhancement for test and fault tolerance will be explored to improve the inference accuracy of ONNs.
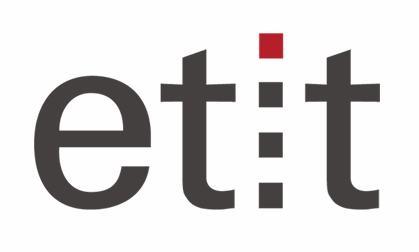