Decoding the Language of Genetics
NLP-Driven Codon Optimization for Enhanced Protein Synthesis Predictions
Master thesis, Bachelor thesis
The aim of this project is to leverage deep learning, specificallyusing a Large Language Model, to identify patterns in homologous proteingene sequences that indicate high expressibility. Using these identified patterns,we aim to predict the producibility of heterologous proteins from their DNAsequences and validate these predictions experimentally.
Background: Microorganism-basedprotein production is a vital part ofindustrial biotechnology, spanningfrom bioethanol-producing enzymesto therapeutic antibodies. A centralchallenge is the efficient synthesisof heterologous (i.e., not native tothe producing organism) proteins.One method to enhance production iscodon optimization, where the DNAsequence is strategically modified tomatch the host organism’s preferredsequence patterns, without changingthe resulting protein. Decodingintricate patterns to achieve adesired output is analogous tonatural language processing (NLP)methods. This topic presents anintersection where informationprocessing techniques meet andaddress biological complexities.
Research Opportunities: We’ve recently developed a Large Language Modelthat operates on amino acid sequences for predicting protein synthesis capability.This model is currently under rigorous evaluation and experimental testing.There are two possibilities for a thesis, one with a computational focus and onewith a biological focus:
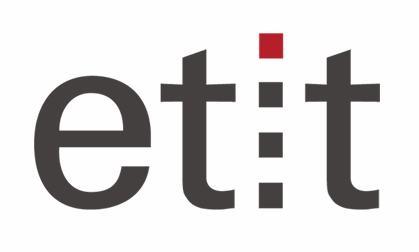