Relaxed continuous time Markov chains
Synthetic Biology / Modeling
Type of work flexible, Master thesis, Bachelor thesis
Deep generative models such as the variational autoencoder have led to breakthroughs in generating synthetic data from complicated distributions, e.g. natural images. The key ingredient of this success story is a new way of parameter learning via sampling-based variational inference.
Continuous time Markov chains (CTMCs) are stochastic processes with discrete states and exponentially distributed waiting times between the jumps. In systems biology, they are routinely used to describe the time evolution of molecule counts within a cell. Due to their discrete nature and the large number of states, learning CTMCs from biological data is still challenging. The goal of this project is to explore relaxation techniques for CTMC simulation. This may pave the way for powerful simulation-based inference techniques and combinations of CTMCs with deep learning architectures.
For further information please look into the PDF or contact the supervisor.
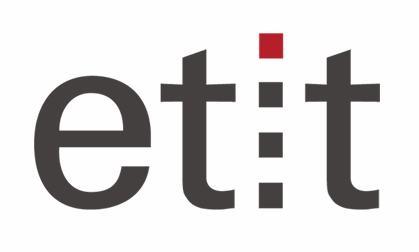